http://www.asyura2.com/14/it12/msg/297.html
Tweet |
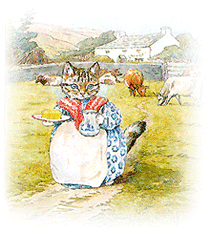
オンラインヘイトの力学
Nature ダイジェスト Vol. 16 No. 12 | doi : 10.1038/ndigest.2019.191233
原文:Nature (2019-09-12) | doi: 10.1038/d41586-019-02447-1 | Strategies for combating online hate
Noemi Derzsy
ソーシャルメディアプラットフォームにおけるオンライン上のヘイトグループの力学の分析から、ヘイトスピーチを禁止する現行のアプローチではうまくいかない理由が明らかになり、オンラインヘイト撲滅に有効な可能性のある4つの戦略の基礎が示された。
metamorworks/iStock / Getty Images Plus/Getty
オンライン上のヘイトグループの生態系は、ソーシャルメディアプラットフォーム上にしぶとく存在し続ける。その仕組みはどのようなものなのか、そして、その存在を効果的に減少させるためにはどんな対策を取ることができるのか? これらの疑問に対し、ジョージ・ワシントン大学(米国ワシントンD.C.)のNeil Johnsonら1は、複数のソーシャルメディアプラットフォームに存在するオンラインヘイトコミュニティの挙動に関して、Nature 2019年9月12日号261ページに興味深い報告をしている。Johnsonらは、オンラインヘイトグループの構造と力学を解明し、その結果を踏まえて、オンラインソーシャルメディア上にはびこるヘイトコンテンツを減らすための4つのポリシーを提案している。
https://www.natureasia.com/ja-jp/ndigest/v16/n12/%E3%82%AA%E3%83%B3%E3%83%A9%E3%82%A4%E3%83%B3%E3%83%98%E3%82%A4%E3%83%88%E3%81%AE%E5%8A%9B%E5%AD%A6/101254
• Letter
• Published: 21 August 2019
Hidden resilience and adaptive dynamics of the global online hate ecology
• N. F. Johnson,
• R. Leahy,
• N. Johnson Restrepo,
• N. Velasquez,
• M. Zheng,
• P. Manrique,
• P. Devkota &
• S. Wuchty
Nature volume 573, pages261–265(2019)Cite this article
Article metrics
• 13k Accesses
• 2 Citations
• 622 Altmetric
• Metricsdetails
Abstract
Online hate and extremist narratives have been linked to abhorrent real-world events, including a current surge in hate crimes1,2,3,4,5,6 and an alarming increase in youth suicides that result from social media vitriol7; inciting mass shootings such as the 2019 attack in Christchurch, stabbings and bombings8,9,10,11; recruitment of extremists12,13,14,15,16, including entrapment and sex-trafficking of girls as fighter brides17; threats against public figures, including the 2019 verbal attack against an anti-Brexit politician, and hybrid (racist–anti-women–anti-immigrant) hate threats against a US member of the British royal family18; and renewed anti-western hate in the 2019 post-ISIS landscape associated with support for Osama Bin Laden’s son and Al Qaeda. Social media platforms seem to be losing the battle against online hate19,20 and urgently need new insights. Here we show that the key to understanding the resilience of online hate lies in its global network-of-network dynamics. Interconnected hate clusters form global ‘hate highways’ that—assisted by collective online adaptations—cross social media platforms, sometimes using ‘back doors’ even after being banned, as well as jumping between countries, continents and languages. Our mathematical model predicts that policing within a single platform (such as Facebook) can make matters worse, and will eventually generate global ‘dark pools’ in which online hate will flourish. We observe the current hate network rapidly rewiring and self-repairing at the micro level when attacked, in a way that mimics the formation of covalent bonds in chemistry. This understanding enables us to propose a policy matrix that can help to defeat online hate, classified by the preferred (or legally allowed) granularity of the intervention and top-down versus bottom-up nature. We provide quantitative assessments for the effects of each intervention. This policy matrix also offers a tool for tackling a broader class of illicit online behaviours21,22 such as financial fraud.
オンラインの憎悪と過激派の物語は、憎悪犯罪の急増1,2,3,4,5,6やソーシャルメディアvitriol7による若者の自殺の驚くべき増加など、忌まわしい現実世界の出来事にリンクされています。クライストチャーチでの2019年の攻撃、刺し、爆撃などの大量射撃の扇動8,9,10,11;過激派の募集12、13、14、15、16、戦闘機の花嫁としての少女の閉じ込めと性的人身売買を含む17。反ブレグジット政治家に対する2019年の口頭攻撃を含む公人に対する脅威、およびハイブリッド(人種差別主義者、反女性、反移民)は、英国王室の米国メンバーに対する脅威を憎む18。そして、オサマビンラディンの息子とアルカイダの支援に関連する2019年のISIS後の状況で反西欧の憎悪を新たにした。ソーシャルメディアプラットフォームは、オンラインでの憎悪との戦いに負けているようであり19、20、新たな洞察が緊急に必要です。ここでは、オンラインヘイトの回復力を理解するための鍵は、グローバルネットワークダイナミクスにあることを示します。相互接続されたヘイトクラスターは、グローバルな「ヘイトハイウェイ」を形成します。これは、集合的なオンライン適応によって支援され、ソーシャルメディアプラットフォームを横断します。私たちの数学モデルは、単一のプラットフォーム(Facebookなど)内でのポリシングが事態を悪化させる可能性があり、最終的にはオンラインでの嫌悪がflour延するグローバルな「ダークプール」を生成すると予測しています。化学における共有結合の形成を模倣する方法で、攻撃時にマイクロレベルで急速に再配線および自己修復する現在のヘイトネットワークを観察します。この理解により、介入の好みの(または法的に許可された)粒度とトップダウン対ボトムアップの性質によって分類された、オンラインの憎悪を打破するのに役立つポリシーマトリックスを提案できます。各介入の効果の定量的評価を提供します。また、このポリシーマトリックスは、金融詐欺などの広範な不正行為21、22に対処するためのツールを提供します。
Main
Current strategies to defeat online hate tend towards two ends of the scale: a microscopic approach that seeks to identify ‘bad’ individual(s) in the sea of online users1,14,16, and a macroscopic approach that bans entire ideologies, which results in allegations of stifling free speech23. These two approaches are equivalent to attempts to try to understand how water boils by looking for a bad particle in a sea of billions (even though there is not one for phase transitions24), or the macroscopic viewpoint that the entire system is to blame (akin to thermodynamics24). Yet, the correct science behind extended physical phenomena24 lies at the mesoscale in the self-organized cluster dynamics of the developing correlations, with the same thought to be true for many social science settings25,26,27.
A better understanding of how the ecology of online hate evolves could create more effective intervention policies. Using entirely public data from different social media platforms, countries and languages, we find that online hate thrives globally through self-organized, mesoscale clusters that interconnect to form a resilient network-of-networks of hate highways across platforms, countries and languages (Fig. 1). Our mathematical theory shows why single-platform policing (for example, by Facebook) can be ineffective (Fig. 2) and may even make things worse. We find empirically that when attacked, the online hate ecology can quickly adapt and self-repair at the micro level, akin to the formation of covalent bonds in chemistry (Fig. 3). We leave a detailed study of the underlying social networks to future work because our focus here is on the general cross-platform behaviour. Knowledge of these features of online hate enables us to propose a set of interventions to thwart it (Fig. 4).
メイン
オンラインの憎悪に打ち勝つための現在の戦略は、スケールの両端に向かう傾向があります:オンラインユーザーの海で「悪い」個人を特定しようとする微視的なアプローチ1,14,16、およびイデオロギー全体を禁止する巨視的なアプローチ言論の自由を抑圧したという主張23。これらの2つのアプローチは、数十億の海で悪い粒子を探して水が沸騰する様子を理解しようとする試みと同等です(相転移24がない場合でも)、またはシステム全体が責任を負うという巨視的な視点(同様)熱力学に24)。しかし、拡張された物理現象の背後にある正しい科学24は、発展している相関関係の自己組織化クラスターダイナミクスの中規模にあり、多くの社会科学設定25、26、27にも同じ考えがあります。
オンラインヘイトの生態がどのように進化するかをよりよく理解することで、より効果的な介入ポリシーを作成できます。さまざまなソーシャルメディアプラットフォーム、国、言語からの完全に公開されたデータを使用すると、プラットフォーム、国、言語をまたがるヘイトハイウェイの回復力のあるネットワークネットワークを相互接続する自己組織化されたメソスケールクラスターを通じて、オンラインヘイトがグローバルに繁栄していることがわかります(図。1)。私たちの数学的理論は、単一プラットフォームのポリシング(たとえばFacebookによる)が効果的でなく(図2)、事態を悪化させる可能性さえあることを示しています。攻撃を受けた場合、オンラインのヘイトエコロジーは、化学における共有結合の形成と同様に、ミクロレベルで迅速に適応および自己修復できることが経験的にわかっています(図3)。ここでの焦点は一般的なクロスプラットフォームの動作にあるため、基礎となるソーシャルネットワークの詳細な研究は今後の作業に任せます。これらのオンライン嫌悪の特徴を知ることで、それを阻止するための一連の介入を提案することができます(図4)。
Fig. 1: Global ecology of online hate clusters.
a, Schematic of resilient hate ecology that we find flourishing online, mixing hate narratives, languages and cultures across platforms. A1, A2 and A3 denote three types of self-organized adaptation that we observe that quickly build new bridges between otherwise independent platforms (see main text). We focus on Facebook (FB) and VKontakte (VK) clusters, shown as large blue and red symbols, respectively; different shapes represent different hate narratives. Undirected (that is, no arrowhead) coloured link between two hate clusters indicates a strong two-way connection. Small black circles indicate users, who may be members of 1, 2, 3…hate clusters; directed (that is, with arrowhead) link indicates that the user is a member of that hate cluster. b, Placing hate clusters at the location of their activity (for example, ‘Stop White Genocide in South Africa’ (SA)) reveals a complex web of global hate highways built from these strong inter-cluster connections. Only the basic skeleton is shown. Bridges between Facebook and VKontakte (for example, A1, A2 and A3 in a) are shown in green. When the focus of a hate cluster is an entire country or continent, the geographical centre is chosen. Inset shows dense hate highway interlinkage across Europe. c, Microscale view of actual KKK hate-cluster ecosystem. The ForceAtlas2 algorithm used is such that the further two clusters are apart, the fewer users they have in common. Hate-cluster radii are determined by the number of members. d, Schematic showing synapse-like nature of individual hate clusters.
a、プラットフォーム全体で憎悪の物語、言語、文化が混ざり合った、回復力のある憎悪生態学の概略図。 A1、A2、およびA3は、それ以外の場合は独立したプラットフォーム間で新しいブリッジを迅速に構築することを確認している3種類の自己組織化適応を示しています(本文参照) Facebook(FB)とVKontakte(VK)クラスターに焦点を当てています。これらはそれぞれ大きな青色と赤色の記号で示されています。さまざまな形は、さまざまな憎悪の物語を表します。 2つのヘイトクラスター間の無向(つまり矢印なし)の色付きリンクは、強力な双方向接続を示します。小さな黒い丸は、1、2、3…憎悪クラスターのメンバーであるユーザーを示します。有向(つまり、矢印付き)リンクは、ユーザーがそのヘイトクラスターのメンバーであることを示します。 b、ヘイトクラスターを活動の場所に配置すると(たとえば、「南アフリカのホワイトジェノサイドを停止」(SA))、これらの強力なクラスター間接続から構築されたグローバルなヘイトハイウェイの複雑な網が明らかになります。基本的なスケルトンのみが表示されます。 FacebookとVKontakteの間のブリッジ(aのA1、A2、A3など)は緑色で表示されます。ヘイトクラスターの焦点が国または大陸全体にある場合、地理的中心が選択されます。挿入図は、ヨーロッパ全体で密集した憎悪高速道路の相互関係を示しています。 c、実際のKKKヘイトクラスターエコシステムのマイクロスケールビュー。使用されるForceAtlas2アルゴリズムでは、さらに2つのクラスターが離れているほど、共通するユーザーが少なくなります。ヘイトクラスター半径は、メンバーの数によって決まります。 d、個々の憎悪クラスターのシナプスのような性質を示す概略図。
Source Data
Full size image
Fig. 2: Mathematical model showing resilience of hate-cluster ecology.
a, Connected hate clusters from Fig. 1a, trying to establish links from a platform such as VKontakte (subset 1b) to a better-policed platform such as Facebook (platform 2), run the risk (cost R) of being noticed by moderators of Facebook and hence sanctions and legal action. Because more links creates more visibility and hence more risk, we assume that the cost of accessing platform 2 from platform 1 is proportional to the number of links, ρ. b, Mathematical prediction from this model (equation (1)) shows that the average shortest path ℓ¯ℓ¯ between hate clusters in VKontakte (subset 1b) has a minimum ℓ¯minℓ¯min as a function of the number of links ρ into platform 2 (Facebook). For any reasonably large number of inter-platform links ρ > ρmin, our theory predicts that the action of platform 2 (such as Facebook) to reduce the number of links ρ will lead to an unwanted decrease in the average shortest path ℓ¯ℓ¯ as ρ decreases towards ρmin. In addition, as the universe of social media expands in the future to many interconnected platforms, as shown schematically in a, our theory predicts that the combined effect of having independent moderators on each platform will be to create spontaneous dark pools of hate (dark region in a).
Full size image
Fig. 3: Adaptive dynamics of online hate at the microscale.
a, The KKK ecosystem on VKontakte before and after the school shooting in Parkland, Florida, on 14 February 2018. During subsequent weeks, rapid microscale rewiring due to individual cluster-joining initiated ‘bonds’ between previously distinct KKK clusters. For clarity, only users (white circles) that change status in the next time step are shown, otherwise the image would be as dense as in b. Larger red nodes are clusters that are closed (that is, closed VKontakte groups), green nodes are open (that is, open VKontakte groups). Yellow links mean the user will leave cluster between day t and day t + 1, meaning that link will disappear. Blue links mean user joins cluster on day t. b, Full KKK ecology on VKontakte after the shooting in Parkland, showing a strong ‘bond’ between the largest KKK clusters. c, Remarkably similar bonding suddenly emerges in anti-western (jihadi) hate-cluster ecology around 18 March 2015, a few days after a coalition strike appears to have wounded ISIS leader Abu Bakr al-Baghdadi. This coincides with rumours immediately circulating among these hate clusters that top ISIS leaders were meeting to discuss who would replace him if he died, suggesting that his injuries were serious. However, none of this become public knowledge in the media—that is, the observed rewiring and self-repair that fuses two clusters into one (that is, two disappeared, shown yellow, and one appeared, shown as blue) is a self-organized, adaptive response of the online hate system. Although b mimics electronic covalent bonding, c is a more extreme version of bonding more akin to nuclear fusion. The ForceAtlas2 algorithm used to plot b and c is such that the further two clusters are apart, the less users they have in common.
Full size image
Fig. 4: Policy matrix from our findings.
Descriptions of policies 1–4 are supplied in the main text, and each policy intervention is shown in green. The best policy for a given setting can be chosen according to the required balance between legally allowed (preferred) granularity and the legally allowed (preferred) nature of intervention.
Full size image
Our analysis of online clusters does not require any information about individuals, just as information about a specific molecule of water is not required to describe the bubbles (that is, clusters of correlated molecules) that form in boiling water. Online clusters such as groups, communities and pages are a popular feature of platforms such as Facebook and VKontakte, which is based in central Europe, has hundreds of millions of users worldwide, and had a crucial role in previous extremist activity27. Such online clusters allow several individual users to self-organize around a common interest27 and they collectively self-police to remove trolls, bots and adverse opinions. Some people find it attractive to join a cluster that promotes hate because its social structure reduces the risk of being trolled or confronted by opponents. Even on platforms that do not have formal groups, quasi-groups can be formed (for example, Telegram). Although Twitter has allowed some notable insights26, we do not consider it here as its open-follower structure does not fully capture the tendency of humans to form into tight-knit social clusters (such as VKontakte groups) in which they can develop thoughts without encountering opposition. Our online cluster search methodology generalizes that previously described27 to multiple social media platforms and can be repeated for any hate topic (see Methods for full details).
The global hate ecology that we find flourishing online is shown in Fig. 1a, b. The highly interconnected network-of-networks28,29,30 mixes hate narratives across themes (for example, anti-Semitic, anti-immigrant, anti-LGBT+), languages, cultures and platforms. This online mixing manifest itself in the 2019 attack in Christchurch: the presumed shooter was Australian, the attack was in New Zealand, and the guns carried messages in several European languages on historical topics that are mentioned in online hate clusters across continents. We uncover hate clusters of all sizes—for example, the hate-cluster distribution for the ideology of the Ku Klux Klan (KKK) on VKontakte has a high goodness-of-fit value for a power-law distribution (Extended Data Fig. 1). This suggests that the online hate ecology is self-organized, because it would be almost impossible to engineer this distribution using top-down control. The estimated power-law exponent is consistent with a sampling of anti-western hate clusters as well as the online ecology of financial fraud21, suggesting that our findings and policy suggestions can help to tackle a broader class of illicit online behaviours21,22.
We observe operationally independent platforms—that are also commercial competitors—becoming unwittingly coupled through dynamical, self-organized adaptations of the global hate-cluster networks. This resilience helps the hate ecology to recover quickly after the banning of single platforms. The three types of adaptation bridging VKontakte and Facebook that enabled hate to re-enter Facebook through the ‘back door’ are shown in Fig. 1a: (A1) hate-cluster mirroring; (A2) hate-cluster reincarnation; and (A3) direct inter-hate-cluster linkage (see Supplementary Information). We observed A2 after Facebook banned the KKK. An ecology of nearly 60 KKK clusters remained on VKontakte (Fig. 1c) that included posts in Ukrainian. When the Ukrainian government banned VKontakte, the VKontakte-based KKK ecosystem (Fig. 1c) reincarnated KKK cluster(s) back on Facebook, but with “KuKluxKlan” written in Cyrillic, making it harder to catch with English-language detection algorithms. Hence, adaptation A2 enabled the hate ideology to implant cluster(s) with thousands of supporters back into a platform in which it was still banned.
A sample of the hate-cluster network placed on a global map using self-reported location information of each cluster is shown in Fig. 1b. This shows how clusters connect across different continents creating one-step highways for hate content. The Facebook and VKontakte hate bridges occur in Europe, the United States and South Africa, even though VKontakte is often thought of as being local to central Europe. Europe (Fig. 1b, inset) shows a particularly complex hate ecology, which reflects intertwined narratives that cross languages and declared causes—for example, neo-Nazi clusters with membership drawn from the United Kingdom, Canada, United States, Australia and New Zealand feature material about English football, Brexit and skinhead imagery while also promoting black music genres. So although the hate may be pure, the rationale given is not, which suggests that this online ecology acts like a global fly-trap that can quickly capture new recruits from any platform, country and language, particularly if they do not yet have a clear focus for their hate.
Our mathematical model in Fig. 2 predicts additional resilience and its negative consequences for the current battle against online hate. It considers the fairly common observation in our data of a ring of c connected hate clusters within a given platform (for example, platform 1, see Extended Data Fig. 2). In our model, each hate cluster is attempting to spread its hate material to other clusters in the ring through links such as A1, A2 and/or A3 (Fig. 1a), but incurs a cost R when its material passes between platforms 1 and 2 because of the risk of sanctions on platform 2 (Facebook is better policed). We assume a probability q of a given hate cluster on platform 1 sending its hate material on a path via platform 2. The following formula, derived in the Supplementary Information, then gives the cluster-averaged value of the shortest path (that is, the average length of the hate highway)30 between the c hate clusters on platform 1:
ℓ¯=R(R−1)2(c−1)+(1−q)c−R[3+q(c−2−R)]q2(c−1)+q[2−2R+2c−q(R−1)(R−c)]−3q2(c−1)ℓ¯=R(R−1)2(c−1)+(1−q)c−R[3+q(c−2−R)]q2(c−1)+q[2−2R+2c−q(R−1)(R−c)]−3q2(c−1)
(1)
Figure 2b shows ℓ¯ℓ¯ as a function of the number of links ρ between platforms 1 and 2 (ρ = cq with c fixed) when R increases linearly with ρ, which is consistent with more links carrying more risk. The minimum in ℓ¯ℓ¯ has an important negative consequence. Suppose platform 2 finds a large number of hate links ρ from 1, and manages to find some and shut them down, hence reducing ρ. It can inadvertently decrease the average shortest path ℓ¯ℓ¯ between hate clusters on platform 1 (for example, VKontakte), hence accelerating how hate content gets shared within platform 1. The existence of several operationally independent platforms (Fig. 2a) with their own moderators and no coordinated cross-platform policing gives rise to a further resilience: our mathematical model (see Supplementary Information) shows that sections of the less policed platforms can then become isolated, creating spontaneous ‘dark pools’ of hate highways (dark region in Fig. 2a).
Further resilience at the micro level occurs in the form of rapid rewiring and self-repair that mimics covalent bonding from chemistry, in apparent response to real-world events (Fig. 3). The ecology of the KKK on VKontakte (Fig. 3a) rewired around accusations just after the school shooting in Parkland, Florida. We do not know of any evidence that these clusters were involved, but news reports discussed the presumed shooter’s interest in the KKK, and its themes and symbols, hence these clusters probably worried about increased scrutiny. Links like chemical bonds quickly form between KKK hate clusters in a bottom-up, self-organized way. This adaptive evolutionary response helps the decentralized KKK ideological organism to protect itself by bringing together previously unconnected supporters. The network is presented on a larger scale in Fig. 3b, with the bonding density of common users clearly visible (white cloud between the green clusters). We also see this same bonding (Fig. 3c) emerge in the response of anti-western jihadist hate groups in 2015 when the leader of the Islamist terrorist group ISIS was reportedly injured in an air strike. We speculate that this covalent bonding is a general adaptive mechanism for online hate, and maybe for other illicit activities.
These insights suggest a matrix of interventions (Fig. 4 and Extended Data Fig. 3) according to the preferred top-down versus bottom-up approach on a given platform and the legal context in a given country. Each policy can be adopted on a global scale simultaneously by all platforms without them needing to share sensitive information. Policy 1 reduces the number of large hate clusters. One might assume that this can be achieved by banning the largest clusters, but the approximate power-law distribution of the size of the hate cluster means that others of similar size will quickly replace them. Instead, policy 1 exploits the underlying self-organizing mechanism by which large clusters form from smaller ones: large clusters can hence be reduced by first banning smaller clusters, with the advantage that smaller clusters are more numerous and easier to find. Policy 2 randomly bans a small fraction of individual users across the online hate population. Choosing a small fraction lowers the risk of multiple lawsuits, and choosing randomly serves the dual role of lowering the risk of banning many from the same cluster, and inciting a large crowd. Policy 3 exploits the self-organized nature of the system by setting clusters against each other in an organic, hands-off way— akin to a human’s immune system. Our data show that there are a reasonable number of active anti-hate users online. Platform managers can encourage anti-hate users to form clusters, for example, through artificial anti-hate accounts as a nucleating mechanism, which then engage in narrative debate with online hate clusters. Online hate-cluster narratives can then be neutralized with the number of anti-hate users determined by the desired time to neutralization. Policy 4 can help platforms with multiple, competing hate narratives. In our data, some white supremacists call for a unified Europe under a Hitler-like regime, and others oppose a united Europe. Similar in-fighting exists between hate clusters of the KKK movement. Adding a third population in a pre-engineered format then allows the hate-cluster extinction time to be manipulated globally (Extended Data Fig. 3).
Limitations to our study include the fact that we cannot yet include all platforms because of a lack of public access. Also, our quantitative analysis is highly idealized in order to generate quantitative answers. Although not intended to capture the complications of any specific real-world setting, the benefit of the modelling approaches in Figs. 2a and 4 is that the output is precisely quantified, reproducible and generalizable, and can therefore help to frame policy discussions as well as probe what-if intervention scenarios. Our findings can also shed light on how illicit networks operate under similar pressures—that is, networks that similarly need to remain open enough to find new recruits yet hidden enough to avoid detection21,22.
これらの洞察は、特定のプラットフォームでのトップダウン対ボトムアップのアプローチと、特定の国の法的状況に応じた介入のマトリックス(図4および拡張データ図3)を示唆しています。各ポリシーは、機密情報を共有する必要なく、すべてのプラットフォームでグローバル規模で同時に採用できます。ポリシー1は、大規模なヘイトクラスターの数を減らします。最大のクラスターを禁止することでこれを達成できると仮定することもできますが、ヘイトクラスターのサイズの近似のべき乗分布は、同様のサイズの他のクラスターがそれらをすぐに置き換えることを意味します。代わりに、ポリシー1は、小さなクラスターから大きなクラスターを形成する基礎となる自己組織化メカニズムを活用します。したがって、最初に小さなクラスターを禁止することにより、大きなクラスターを減らすことができ、小さなクラスターがより多く、より見つけやすいという利点があります。ポリシー2は、オンラインの憎悪人口全体の個々のユーザーのごく一部をランダムに禁止します。小さな割合を選択すると、複数の訴訟のリスクが低下します。ランダムに選択すると、同じクラスターから多くの人を禁止するリスクを軽減し、大勢の人を扇動するという二重の役割を果たします。ポリシー3は、人間の免疫システムに似た、有機的で人手を介さずにクラスターを相互に設定することにより、システムの自己組織化された性質を活用します。私たちのデータは、オンラインでアクティブなアンチヘイトユーザーがかなりの数いることを示しています。プラットフォーム管理者は、たとえば、中核化メカニズムとしての人工的な憎悪防止アカウントを通じて、憎悪防止ユーザーがクラスターを形成することを奨励し、オンライン憎悪クラスターとの物語の議論に従事することができます。その後、オンラインのヘイトクラスターのナラティブを中和することができます。これには、中和するまでの時間によって決まるアンチヘイトユーザーの数を使用します。ポリシー4は、複数の競合する憎悪の物語を持つプラットフォームを支援できます。私たちのデータでは、一部の白人至上主義者は、ヒトラーのような体制の下で統一されたヨーロッパを要求し、他は統一されたヨーロッパに反対しています。 KKK運動の憎悪集団の間にも同様の戦闘が存在します。事前に設計された形式で3番目の集団を追加すると、ヘイトクラスターの消滅時間をグローバルに操作できるようになります(拡張データ図3)。
私たちの研究の制限には、パブリックアクセスが不足しているためにすべてのプラットフォームを含めることができないという事実が含まれます。また、定量分析は、定量的な回答を生成するために非常に理想化されています。特定の現実世界の設定の複雑さをキャプチャすることを意図したものではありませんが、図1と図2のモデリングアプローチの利点は、 2aおよび4は、出力が正確に定量化され、再現性があり、一般化可能であるため、ポリシーの議論を組み立て、what-if介入シナリオを調査するのに役立ちます。私たちの調査結果は、同様のプレッシャーの下で不正ネットワークがどのように機能するか、つまり、同様に新しい新兵を見つけるのに十分にオープンでありながら、検出を回避するのに十分に隠れている必要があるネットワークに光を当てることができます21,22
Methods
Our online cluster search methodology is a direct generalization of that previously described27, but now looking at several social media platforms. It can be repeated for any hate topic, but we focus here on extreme right-wing hate because it is prevalent globally and has been linked to many recent violent real-world attacks. We observe many different forms of hate that adopt similar cross-platform tricks. Whether a particular cluster is strictly a hate philosophy, or simply shows material with tendencies towards hate, does not alter our main findings. Our research avoids the need for any information about individuals, just as information about a specific molecule of water is not needed to describe the bubbles (that is, clusters of correlated molecules) that form in boiling water. Our hate-cluster network analysis starts from a given hate cluster ‘A’ and captures any hate cluster ‘B’ to which hate cluster A has shared an explicit cluster-level link, and vice versa from B to A (see Supplementary Information).
We also developed software to perform this process automatically and, after cross-checking the findings with our manual list, were able to obtain approximately 90% consistency between the manual and automated versions. Each day, we iterated this process until the search led back to hate clusters that were already in the list. For the global hate network, we identified 768 nodes (that is, hate clusters) and 578 edges. This is larger than the number of clusters obtained in the previous study27 of anti-western hate (specifically, pro-ISIS aggregates, which numbered a few hundred on VKontakte). But the fact it is of similar magnitude suggests that the process by which billions of users cluster globally online into hate communities is such that it produces hundreds of clusters—not tens of thousands but also not ten or so.
Although we observe some hate clusters with connections to clusters outside such a subset, these tend to lead down rabbit holes into pornography and other illicit material, so we ignore them. Hence, although this is ‘big data’ in terms of there being approximately 1 million hate-driven individuals globally, the number of clusters into which they form is rather small. For the global hate-cluster network, the numbers of each type of link and node (cluster) are as follows. For the edges, Facebook–Facebook = 64 (11.1%); Facebook–VKontakte = 12 (2.1%); VKontakte–VKontakte = 502 (86.8%). For the nodes (clusters): Facebook = 26 (3.4%); VKontakte = 742 (96.6%). For the example subset on the world map in Fig. 1b: for the edges, Facebook–Facebook = 36 (35.3%); Facebook–VKontakte = 6 (5.9%); VKontakte–VKontakte = 60 (58.8%). For the nodes: Facebook = 14 (26.9%); VKontakte = 38 (73.1%). The details behind Fig. 2 are provided in the Supplementary Information and build on the previous study30. For the calculations of policy modelling (see Supplementary Information), our results in Extended Data Fig. 3 were generated for populations of size 1,000–10,000, but similar results and conclusions emerge for any large number: the calculated effects of policies 1–4 are universal in that they do not depend on the specific numbers chosen. For just the KKK cluster dataset on VKontakte in Figs. 1c and 3, there are 50–60 distinct clusters at any one time, with a total of around 10,000 individuals from across the globe as followers. We include an explicit study of KKK clusters purely because KKK ideology is classified as hate by the Anti-Defamation League and the Southern Poverty Law Center. Its unique name and well-defined symbols make it easy to classify. Whether a particular cluster is strictly a hate philosophy, or instead shows material with tendencies towards hate, does not alter our main findings. The largest cluster has just over 10,000 followers and the smallest has fewer than 10, hence there is a very broad distribution. As shown in Extended Data Fig. 1a, the distribution of cluster sizes (that is, the number of followers) is consistent with a power law with a very high goodness-of-fit value of P = 0.92.
Reporting summary
Further information on research design is available in the Nature Research Reporting Summary linked to this paper.
Data availability
The dataset is provided as Source Data. The open-source software packages Gephi and R were used to produce the networks in the figures. No custom software was used.
References
1. 1.
The UK Home Affairs Select Committee. Hate Crime: Abuse, Hate and Extremism Online. session 2016–17 HC 609 https://publications.parliament.uk/pa/cm201617/cmselect/cmhaff/609/609.pdf (2017).
2. 2.
Patrisse Cullors. Online Hate Is A Deadly Threat https://edition.cnn.com/2018/11/01/opinions/social-media-hate-speech-cullors/index.html (2017).
3. 3.
Beirich, H., Hankes, K., Piggott, S., Schlatter, E. & Viets, S. The Year in Hate and Extremism https://www.splcenter.org/fighting-hate/intelligence-report/2017/year-hate-and-extremism (2017).
4. 4.
Hohmann, J. Hate Crimes Are a Much Bigger Problem than Even the New FBI Statistics Show https://www.washingtonpost.com/news/powerpost/paloma/daily-202/2018/11/14/daily-202-hate-crimes-are-a-much-bigger-problem-than-even-the-new-fbi-statistics-show/5beba5bd1b326b39290547e2/?utm_term=.e203814306e8 (2018).
5. 5.
Reitman, J. U.S. Law Enforcement Failed to See the Threat of White Nationalism. Now they Don’t Know How to Stop It https://www.nytimes.com/2018/11/03/magazine/FBI-charlottesville-white-nationalism-far-right.html (2018).
6. 6.
Southern Poverty Law Center (SPLC). Extremist Groups https://www.splcenter.org/fighting-hate/extremist-files/groups (2018).
7. 7.
John, A. et al. Self-harm, suicidal behaviours, and cyberbullying in children and younG people: systematic review. J. Med. Internet Res. 20, e129 (2018).
o Article
o Google Scholar
8. 8.
Berman, M. Prosecutors Say Accused Charleston Church Gunman Self-Radicalized Online https://www.washingtonpost.com/news/post-nation/wp/2016/08/22/prosecutors-say-accused-charleston-church-gunman-self-radicalized-online/?utm_term=.4f17303dffd4 (2016).
9. 9.
Pagliery, J. The Suspect in Congressional Shooting Was Bernie Sanders Supporter, Strongly Anti-Trump http://www.cnn.com/2017/06/14/homepage2/james-hodgkinson-profile/index.html (2017).
10. 10.
Yan, H., Simon, D. & Graef, A. Campus Killing: Suspect is a Member of ‘Alt-Reich’ Facebook Group http://www.cnn.com/2017/05/22/us/university-of-maryland-stabbing/index.html (2017).
11. 11.
Amend, A. Analyzing a Terrorist’s Social Media Manifesto: the Pittsburgh Synagogue Shooter’s Posts on Gab https://www.splcenter.org/hatewatch/2018/10/28/analyzing-terrorists-social-media-manifesto-pittsburgh-synagogue-shooters-posts-gab (2018).
12. 12.
Gill, P. & Corner, E. in Terrorism Online: Politics, Law, Technology (eds Jarvis, L. et al.) Ch. 1 (Routledge, 2015).
13. 13.
Gill, P. et al. Terrorist use of the internet by the numbers quantifying behaviors, patterns, and processes. Criminol. Public Pol. 16, 99–117 (2017).
o Google Scholar
14. 14.
Gill, P. Lone Actor Terrorists: A Behavioral Analysis (Routledge, 2015).
15. 15.
Gill, P., Horgan, J. & Deckert, P. Bombing alone: tracing the motivations and antecedent behaviors of lone-actor terrorists. J. Forensic Sci. 59, 425–435 (2014).
o Article
o Google Scholar
16. 16.
Schuurman, B. et al. End of the lone wolf: the typology that should not have been. Stud. Conflict Terrorism 42, 771–778 (2017).
o Article
o Google Scholar
17. 17.
Panin, A. & Smith, L. Russian Students Targeted as Recruits by Islamic State https://www.bbc.co.uk/news/world-europe-33634214 (2015).
18. 18.
Foster, M. The Racist Online Abuse of Meghan Markle Has Put Royal Staff on High Alert https://www.cnn.com/2019/03/07/uk/meghan-kate-social-media-gbr-intl/index.html (2019).
19. 19.
Wakefield, J. Christchurch Shootings: Social Media Races to Stop Attack Footage https://www.bbc.com/news/technology-47583393 (2019).
20. 20.
O’Brien, S. A. Moderating the Internet is Hurting Workers https://www.cnn.com/2019/02/28/tech/facebook-google-content-moderators/index.html (2019).
21. 21.
KrebsOnSecurity. Deleted Facebook Cybercrime Groups Had 300,000 Members https://krebsonsecurity.com/2018/04/deleted-facebook-cybercrime-groups-had-300000-members/ (2019).
22. 22.
Wong, J. C. Anti-Vaxx Mobs: Doctors Face Harassment Campaigns on Facebook https://www.theguardian.com/technology/2019/feb/27/facebook-anti-vaxx-harassment-campaigns-doctors-fight-back (2019).
23. 23.
Martínez, A. G. Want Facebook to Censor Speech? Be Careful What You Wish For https://www.wired.com/story/want-facebook-to-censor-speech-be-careful-what-you-wish-for/ (2018).
24. 24.
Stanley, H. E. Introduction to Phase Transitions and Critical Phenomena (Oxford Univ. Press, 1988).
25. 25.
Hedström, P., Sandell, R. & Stern, C. Meso-level networks and the diffusion of social movements. Am. J. Sociol. 106, 145–172 (2000).
o Article
o Google Scholar
26. 26.
Grinberg, N., Joseph, K., Friedland, L., Swire-Thompson, B. & Lazer, D. Fake news on Twitter during the 2016 U.S. presidential election. Science 363, 374–378 (2019).
o ADS
o CAS
o Article
o Google Scholar
27. 27.
Johnson, N. F. et al. New online ecology of adversarial aggregates: ISIS and beyond. Science 352, 1459–1463 (2016).
o ADS
o CAS
o Article
o Google Scholar
28. 28.
Havlin, S., Kenett, D. Y., Bashan, A., Gao, J. & Stanley, H. E. Vulnerability of network of networks. Eur. Phys. J. Spec. Top. 223, 2087 (2014).
o Article
o Google Scholar
29. 29.
Palla, G., Barabási, A. L. & Vicsek, T. Quantifying social group evolution. Nature 446, 664–667 (2007).
o ADS
o CAS
o Article
o Google Scholar
30. 30.
Jarrett, T. C., Ashton, D. J., Fricker, M. & Johnson, N. F. Interplay between function and structure in complex networks. Phys. Rev. E 74, 026116 (2006).
o ADS
o MathSciNet
o Article
o Google Scholar
Download references
Acknowledgements
N.F.J. is supported by US Air Force (AFOSR) grant FA9550-16-1-0247.
Author information
Affiliations
1. Physics Department, George Washington University, Washington, DC, USA
o N. F. Johnson
o , R. Leahy
o & N. Johnson Restrepo
2. Elliot School of International Affairs, George Washington University, Washington, DC, USA
o N. Velasquez
3. Physics Department, University of Miami, Coral Gables, FL, USA
o M. Zheng
o & P. Manrique
4. Computer Science Department, University of Miami, Coral Gables, FL, USA
o P. Devkota
o & S. Wuchty
Contributions
All authors contributed to the research design, the analysis and writing the paper.
Corresponding author
Correspondence to N. F. Johnson.
Ethics declarations
Competing interests
The authors declare no competing interests.
Additional information
Publisher’s note: Springer Nature remains neutral with regard to jurisdictional claims in published maps and institutional affiliations.
Peer review information Nature thanks Paul Gill, Nour Kteily and the other, anonymous, reviewer(s) for their contribution to the peer review of this work.
Extended data figures and tables
Extended Data Fig. 1 Power laws.
a, b, Power laws for the KKK ecology (a) and the ecology of illicit financial activities (b). Their power-law exponents (α) are similar in a and b, and also consistent with c. c, The results from aggregating data from different thematic subsystems, each of which has a power-law distribution with an exponent (βi) distributed around 2.5. d, Summary of the simulation procedure. N power-law distributions are created with a power-law exponent distributed around 2.5. Power-law exponents were then sampled, followed by a power-law test. e, Distribution of the resulting power-law exponents from this simulation procedure, for different values of the mean number of points in the underlying distributions (mu values). The resulting power law exponents α are centred near 1.7, as observed in a and b.
Extended Data Fig. 2 Cluster loop.
a, Cluster loop from Fig. 2. b, Example of a loop of clusters.
Extended Data Fig. 3 Predicted policy effects.
a, The effects of policy 1, with on average more than 550 widely spaced time steps for τ = 10 and N = 104. If the size of an aggregate remains within the range smin to smax for a particular time period τ, that aggregate then fragments. b, The effects of policy 2. Colours represent different intervention starting times (tI) in units of days (vertical grey lines): green tI = 80, red tI = 120, blue tI = 200. Line types represent different percentages of individuals randomly removed (that is, banned) at time tI: dashed line 10%, dotted line 30%, solid line 50%. c, Results for policy 3 of the time to extinction (T) as a function of the initial population partition (N + P = 1,000 fixed, with N being the initial size of the hate population and P being the initial size of the anti-hate population) and the banning rate of the platform, from numerical simulations and also analytic theory. d, Policy 4 shows effect of different allocations of 100 peacekeepers in the hate-cluster versus anti-hate-cluster scenario. nc is the number of clusters of peacekeepers (that is, individuals of type C) that have size sc.
Supplementary information
Supplementary Information
Supplementary Methods, Supplementary Discussion, Supplementary Equations and Supplementary Notes.
Reporting Summary
Source data
Source Data Fig. 1
https://www.nature.com/articles/s41586-019-1494-7
投稿コメント全ログ コメント即時配信 スレ建て依頼 削除コメント確認方法

すべてのページの引用、転載、リンクを許可します。確認メールは不要です。引用元リンクを表示してください。